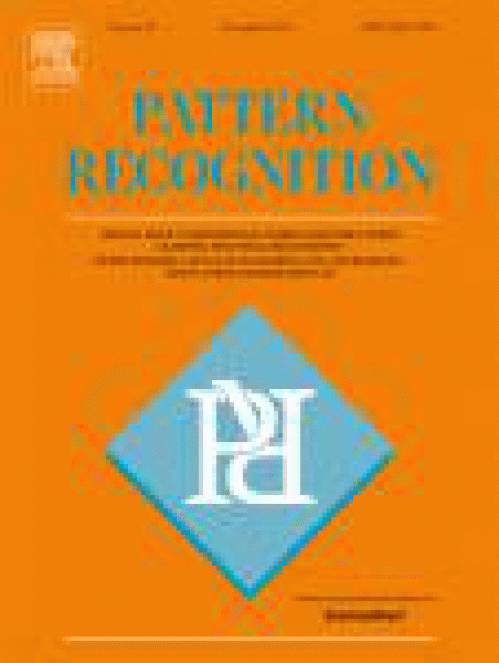
عنوان انگلیسی: Class relatedness oriented-discriminative dictionary learning for multiclass image classification
سال نشر: ۲۰۱۶
نویسنده: Dongyu Zhang,Pengju Liu,Kai Zhang,Hongzhi Zhang,Qing Wang,Xiaoyuan Jing
تعداد صفحه فارسی: 14– تعداد صفحه انگلیسی: ۸
دانشگاه: Sun Yat-Sen University, Guangzhou 510275, China b Harbin Institute of Technology, Harbin 150001, China c State Key Laboratory of Software Engineering of Wuhan University, WuHan 430079, China
نشریه: Process Safety and Environmental Protection
کیفیت ترجمه: ترجمه پلاس
چکیده
یادگیری واژه نامه (DL) اخیراً به شدت مورد توجه قرار گرفته است که به علت قدرت قابل توجه و متمایزش در طبقه بندی امورات و کارهای مختلف می باشد. با وجود اینکه پیشرفت های زیادی در روش های DL به وجود آمده است، اما هنوز هم یک مشکل واضح وجود دارد که چطور می توان یک رابطه را بین اجزای واژه نامه و برچسب های کلاس در طبقه بندی ایجاد کرد. در این مقاله، فرض بر این است که ارتباط بین اجزای یک واژه نامه می توانند در طبقه بندی مولتی کلاس مفید باشند و ما یک رابطه طبقاتی (CRO) را بر پایه یادگیری واژه نامه ارائه کرده ایم. با استفاده از نورم ℓ۱;۱ در کدبندی ضرایب برنامه نویسی، می توان از یک روش پیشنهادی انطباقی برای ایجاد ارتباط بین اجزای واژه نامه و برچسب های مولتی کلاس ایجاد کرد. نتایج تجربی در تشخیص چهره، طبقه بندی اشیاء و شناخت فعالیت نشان می دهد که روش پیشنهادی ما قابل مقایسه با بسیاری از روش های DDL در حوزه هنری می باشد.
Abstract
Dictionary learning (DL) has recently attracted intensive attention due to its representative and discriminative power in various classification tasks. Although much progress has been reported in the existing supervised DL approaches, it is still an open problem that how to build the relationship between dictionary atoms and the class labels in multiclass classification. In this paper, based on the assumption that the relevance of dictionary atoms could be helpful in multiclass classification task, we proposed a class relatedness oriented (CRO) discriminative dictionary learning method for sparse coding. Utilizing the -norm regularization on the coding coefficient matrix, the proposed method can adaptively learn the class relatedness between dictionary atoms and the multiclass labels. Experimental results of face recognition, object classification, and action recognition demonstrate that our proposed method is comparable to many state-of-the-art DDL methods
vfiii3jp4hg5carj8gx97qj5w3u9u8jhicxcptq9_1678189161_59450_11581_1035.zip3.08 MB |